CASE STUDY
Redbubble: Introducing automated content moderation for a global online marketplace
Building a greater digital retail experience with machine learning.
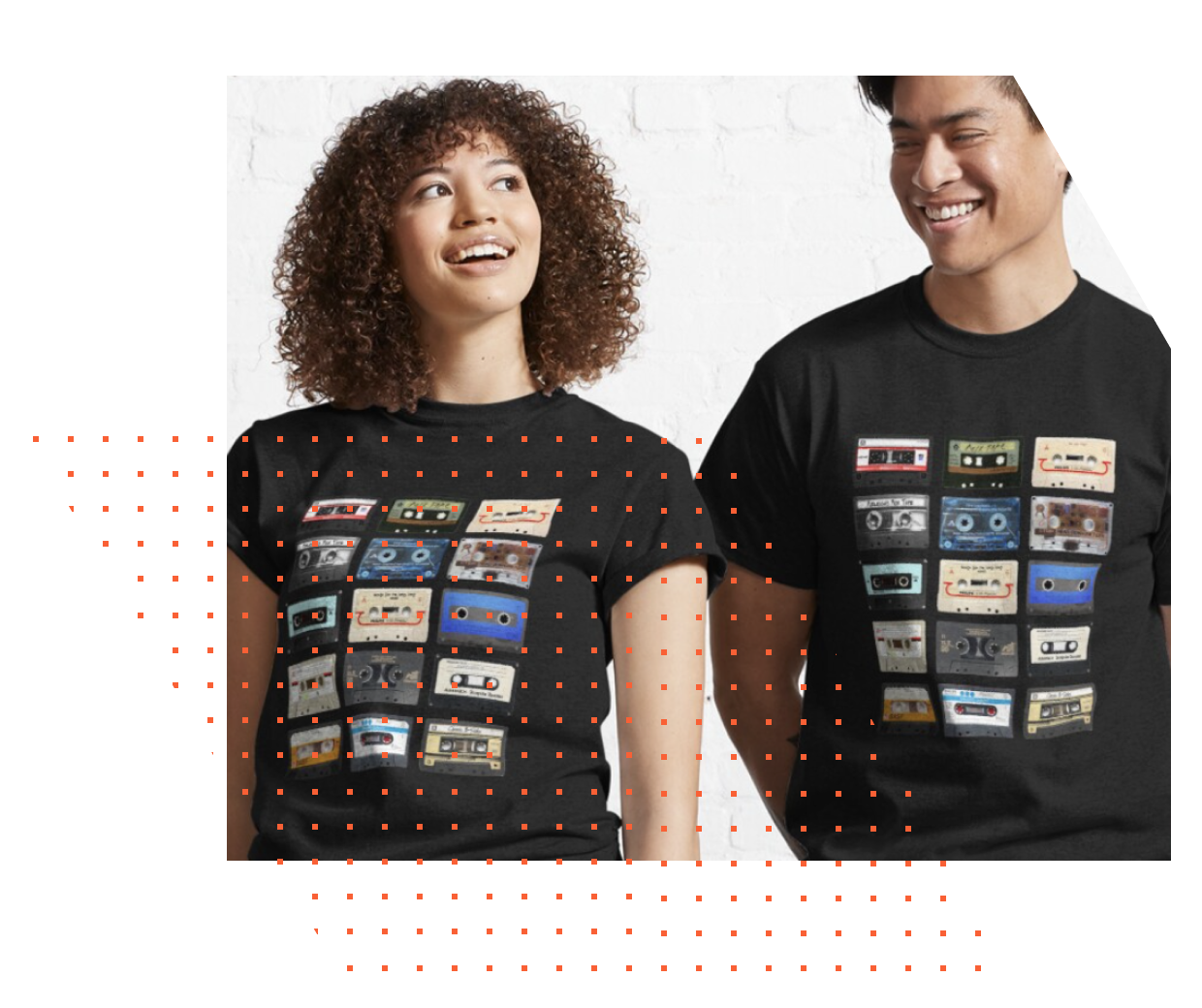
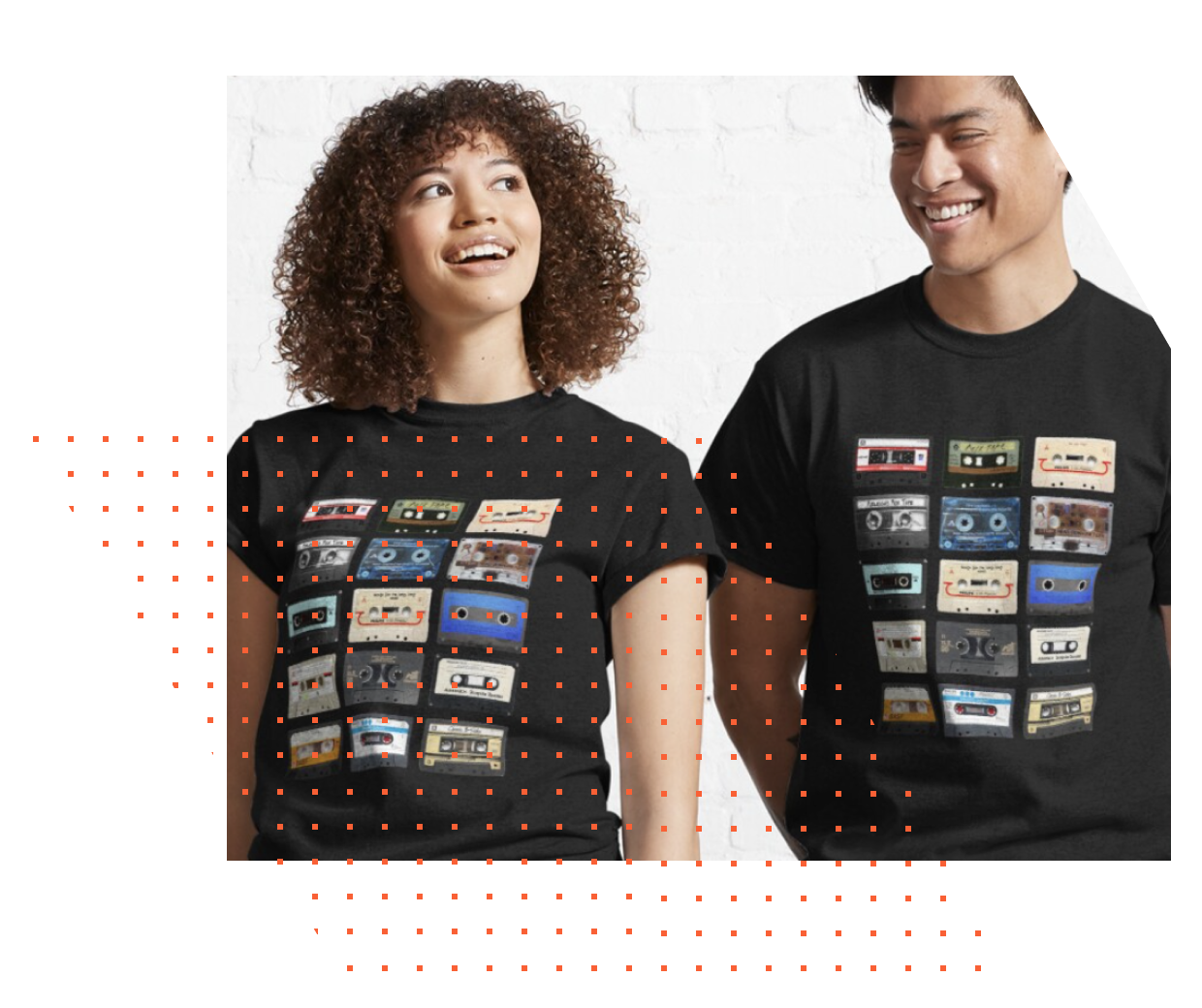
At a glance
DiUS partnered with Redbubble to provide additional skills and support during a huge period of increased demand on the marketplace platform. We created a content moderation solution that utilises machine learning capabilities to moderate the use of copyrighted and offensive material with approximately 80% accuracy, freeing up its team to work on other important parts of the business.
Our services:
Meet Redbubble
Redbubble is a global online marketplace for print-on-demand products based on user-submitted artwork. The company was founded in 2006 in Melbourne and also maintains offices in San Francisco and Berlin.
Redbubble operates primarily on the internet and allows its members to sell their artwork as decoration on a variety of products. Products include prints, T-shirts, hoodies, cushions, duvet covers, leggings, stickers, skirts, and scarves. The company offers free membership to artists who maintain the copyrights to their work, regulate their own prices, and decide which products may display their images.
It hosts a three‑sided global online marketplace, where independent artists upload their designs and creative works for sale on a range of products. When a customer places an order, the product is produced on demand and shipped by a fulfillment partner within Redbubble’s global third-party supply network.
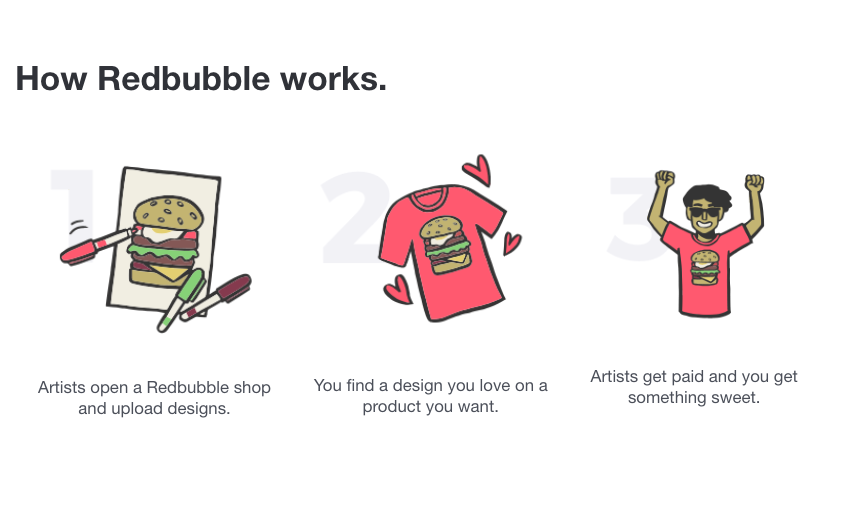
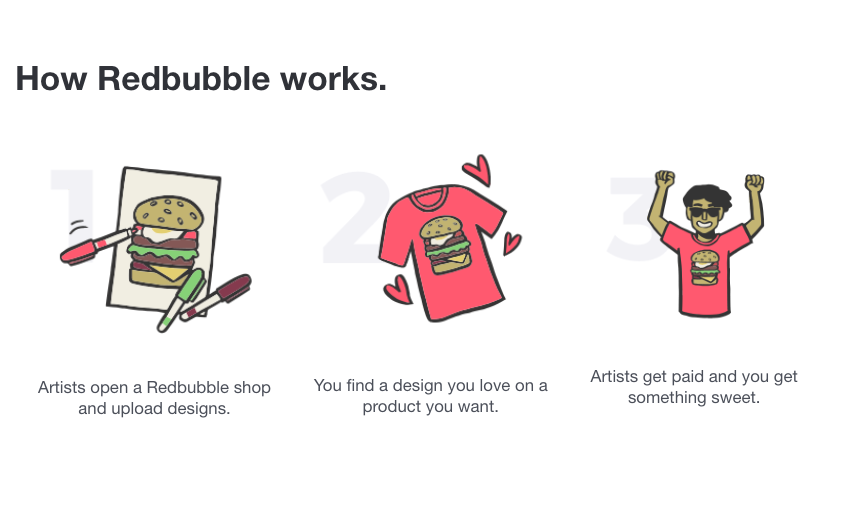
The challenge
Keeping up with huge growth in print-on-demand products
While many organisations faced significant downturns during Covid-19 lockdowns, Redbubble experienced a greater demand in online shopping, as well as an increase in artists using their downtime to create more artwork to sell through the Redbubble marketplace.
After a review, the team needed urgent capability uplift within the organisation, especially around vetting materials coming onto the platform to ensure they weren’t offensive or inappropriate and that they followed trademark guidelines. This was a manual process that was very labour intensive and under greater pressure with the increased demand.
Historically, a team of human content reviewers had been reviewing and vetting the content that was being uploaded to the platform. The amount of content being added on a daily basis had been surging and the human review team was not able to keep up with the load. Therefore, utilising Machine Learning to automate the content review process was put on the roadmap.
What we did
Using computer vision to automate manual processes
DiUS explored various options to build an end-to-end machine learning solution that would automatically detect unauthorised copyrighted logos, as well as inappropriate and offensive language or images. Detecting logos by itself is a classic object detection problem within the computer vision domain, but sometimes there is more to the problem than just simply detecting a logo. The fact that some copyrighted logos are referenced in some artworks does not necessarily mean that a particular artwork is infringing.
Some artists reference and tease brands while transforming them visually to an extent that makes the artwork non-infringing. It is then up to the human reviewer to make the call as to whether a reference of a copyrighted logo is “transformative” enough or not. This makes moderating the content in such a scenario really tricky and subjective.
A Proof of Concept was established to compare two off-the-shelf products. For this particular use case, Visua was chosen to detect imagery that could be considered offensive. The team has since been working on a text detection solution so that both imagery and text can be automatically reviewed together. DiUS was a big part of designing and implementing the image recognition solution and rolling it out to production. Here is a summary of what DiUS did:
Technical assessment and high-level design
The implementation of the third-party solution was carried out by first assessing the existing tech stack and learning about the technical limitations.
Detailed solution design
We had to take into account a few major considerations for coming up with the final version of the detailed design, the most important of which was the ability to cope with the load of constantly incoming artworks and their associated images that needed to be processed. Moreover, we had to make sure our design was scalable in the sense that it would allow adding other vetting mechanisms like text processing later on.
Managing interactions with the third-party service provider
An important part of designing and implementing the image recognition solution was to provide the baseline data to VISUA to train its machine learning models for future predictions. DiUS developed scripts and managed collaborations with the VISUA team to make sure the training data is prepared in the cleanest and most usable way possible and also drove brainstorming sessions with VISUA to tackle challenges along the way.
Implementation and rollout
Software and ML consultants at DiUS were in charge of implementing the back-end image recognition solution as well as the front-end components of the moderation portal in collaboration with other engineering teams from Redbubble.
Following the success of this content moderation system, the team is now implementing a similar approach on the use of trademarks and the adherence of trademark guidelines.


Results for Redbubble
Transforming at speed and scale
DiUS was able to provide the capability and resource uplift that Redbubble urgently needed during a dramatic shift in its business. We introduced new ways of working, helped establish new teams, and provided thought leadership by introducing automated computer vision approaches to managing content.
As per our initial assessments, the infringing image ratio was five in 1,000, meaning that in every 1,000 images, roughly five contained infringing use of copyrighted content. Given that Redbubble was already relying on a human review team of 60 reviewers, scaling up the manual review process carried out by humans was not feasible. By integrating the automated ML-based solution, Redbubble was able to increase the accuracy in detecting infringing content, which lowers the risk for potential legal challenges in the future.